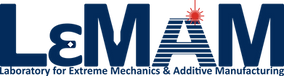
Laboratory for Extreme Mechanics & Additive Manufacturing
Zou's Research Group | Department of Material Science and Engineering
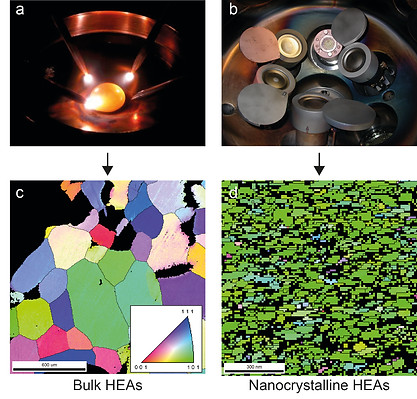
Sample preparation of HEAs: (a) the bulk coarse-grained HEAs produced by arc melting and (c) the inverse pole figure map of the bulk HEAs; (b) the thin-film nanocrystalline (<100 nm grain size) HEAs produced by the magnetron co-sputtering system equipped with four targets arranged in a symmetry, and (d) their inverse pole figure map showing texture.
-
Y. Zou, et al. “Nanocrystalline high entropy alloys: A new paradigm in high temperature strength and stability” Nano Letters (2017))
-
Y. Zou, et al. “Ultrastrong, ductile and stable high-entropy alloys at small scales” Nature Communications 6 (2015).
-
Y. Zou, et al., “Size-dependent plasticity in an Nb25Mo25Ta25W25 refractory high-entropy alloy” Acta Materialia (2014)
Metallic Materials
Design of high-strength and thermally stable alloys using novel fabrication and testing techniques.
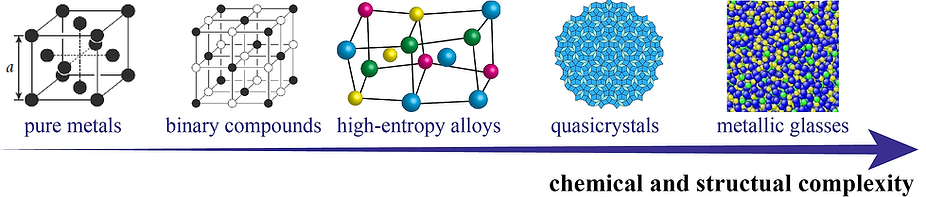
Study structure-property relationship from Simple Ionic Crystals to Complex Intermetallic Phases
Multiscale Mechanics
-
Mechanics: multiscale mechanics, in-situ instrumentation, and high strain-rate/temperature deformation
-
More details coming soon...
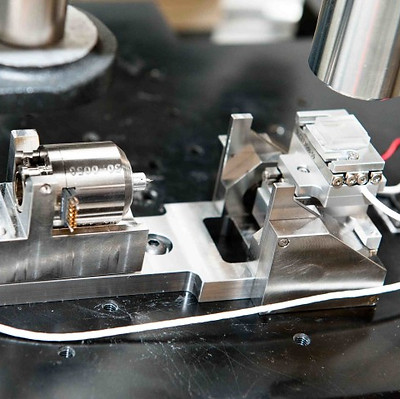
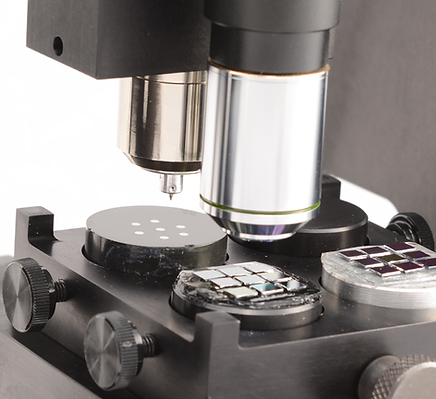
SEM in situ nanomechanical testing system (left) and standalone nanoindenter system (right)
Additive Manufacturing
-
Manufacturing: metal additive manufacturing (3D printing), cold spray technology, powder metallurgy, and micro- and nanofabrication
-
More details coming soon...
_JPG.jpg)
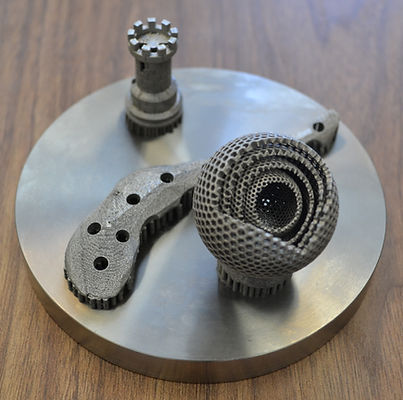_JPG.jpg)
Steel (left) and titanium (right) samples produced by additive manufacturing
Machine Learning for alloy design and processing optimization
-
Machine Learning: The rapid development of artificial intelligence (AI), intelligent methods have been widely applied in materials science research and manufacturing processes. Our group is seeking novel approaches to use AI for material discovery and the optimization of manufacturing processes, particularly on machine learning for mechanics of materials, alloy design, topological/microstructure optimization, and in-situ monitoring.
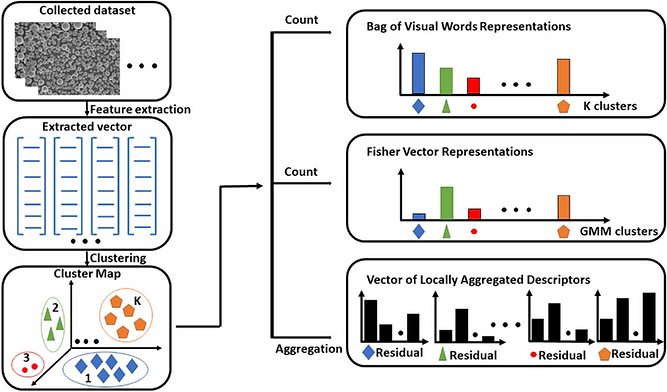
A computer vision approach to evaluate powder flowability for metal additive manufacturing

Additive manufacturing process monitoring : (a) experimental setup (b) our proposed vanilla model (c) class-discriminative maps of the melt pool
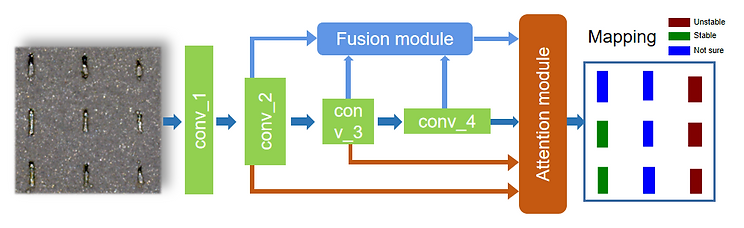
Detection and classification deep learning model for the melt tracks